According to the 2023 data from the Ministry of Health and Welfare in Taiwan, tumors on the lung, breast, liver, kidney, and lymph were very likely to become brain metastases and were malignancies despite that the brain tumor was not on the top 10 list of the cancer mortality; therefore, the follow-up treatment on brain tumors has become the focus of the global healthcare recently. Among all the brain tumor cases, two types of primary tumors, meningioma and acoustic neuroma, plus metastases accounted for 80%. In addition, those cases mostly applied non-invasive radiotherapy and depended on the contouring accuracy of preoperative tumor annotation, positioning, and measuring, resulting in substantially affecting the therapeutic effectiveness.
The tumor number of metastases differs from that of meningioma and acoustic neuroma, from a number of metastases to dozens of metastases to even hundreds of metastases. To determine the volume, size, and location of each metastasis, it has to rely on radiologists to manually review many images of different cross-sections of the two-dimensional (2D) Magnetic Resonance Imaging (MRI). Besides, the manpower of radiologists in hospitals is often short-staffed, which results in patients having to wait on tenterhooks for two to three weeks for the primary MRI scanning result report, leading to missing the best opportunity for early treatment.
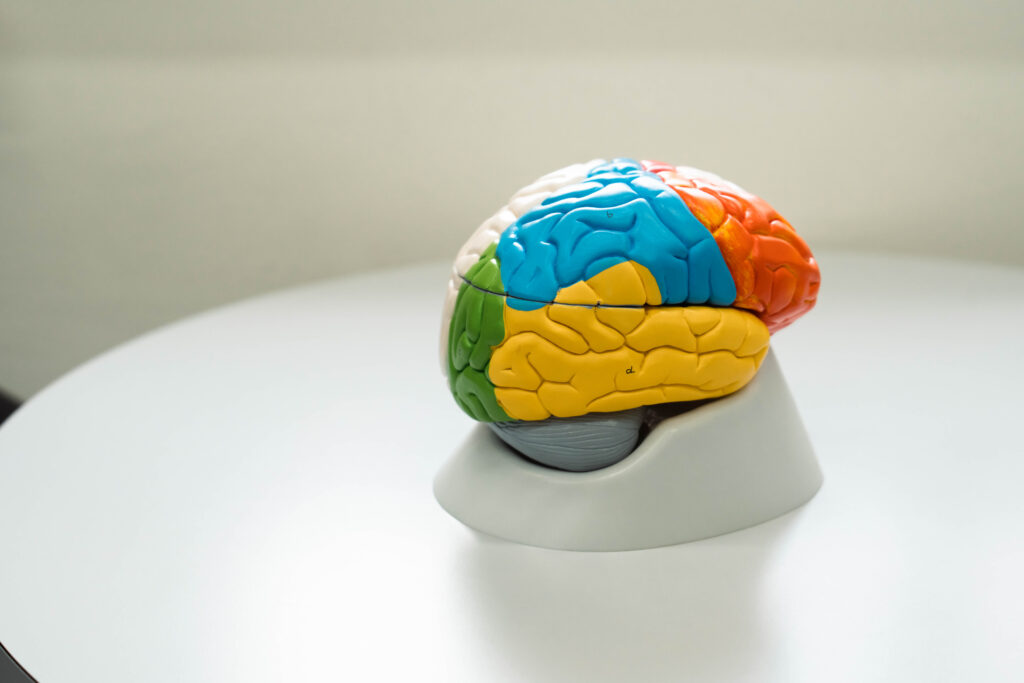
“As far as I know, the radiologists in certain major hospitals have to output 70 to even 100 imaging examination and interpretation reports per person per day. Their working loading is tremendous,” said Dr. Yu-Te Wu, the Deputy Dean at the College of Biomedical Science and Engineering and the Distinguished Professor of the Institute of Biophotonics at National Yang Ming Chiao Tung University (NYCU).
“Just as the Global Navigation Satellite System can plan the closest driving route for drivers to reach the destination and reduce the possibility of ‘drowsy driving’, I have been wondering about how to characterize AI as a navigator in the course of brain tumor diagnosis to assist physicians to complete most of the brain annotation tasks. Physicians will not miss any tumors because of “drowsy visiting”; therefore, patients can receive the most precise treatment regime as quickly as possible,” indicated Dr. Wu.
Millions of MRI Images for A Comprehensive Training Model
To resolve the enormous working loading of marking brain tumors by physicians and return to the diagnostics course centralized on “patients’ needs”, Dr. Wu, who has devoted to Brain Science and Artificial Intelligence in Biomedical Image fields for more than 20 years, has led many teachers and students in the master and doctoral program and multidisciplinary fields of Medicine, Biomedical Imaging and Radiological Sciences, Biomedical Engineering, Electronics and Electrical Engineering, Physics, and Chemistry, etc. to collaborate with the Taipei Veterans General Hospital on acoustic neuroma related AI program of the Ministry of Science and Technology (MOST) Project of “Establishment of Tremendous Data and Applied Research Project for Medical Imaging “ from 2018. The model developed under the MOST AI Project, also sponsored by the Taiwan Germination Program in 2022 and 2023, eventually, was successfully produced “AItewan DeepBT Detector” in 2023 with its overall research and development costs over millions of USD dollars.
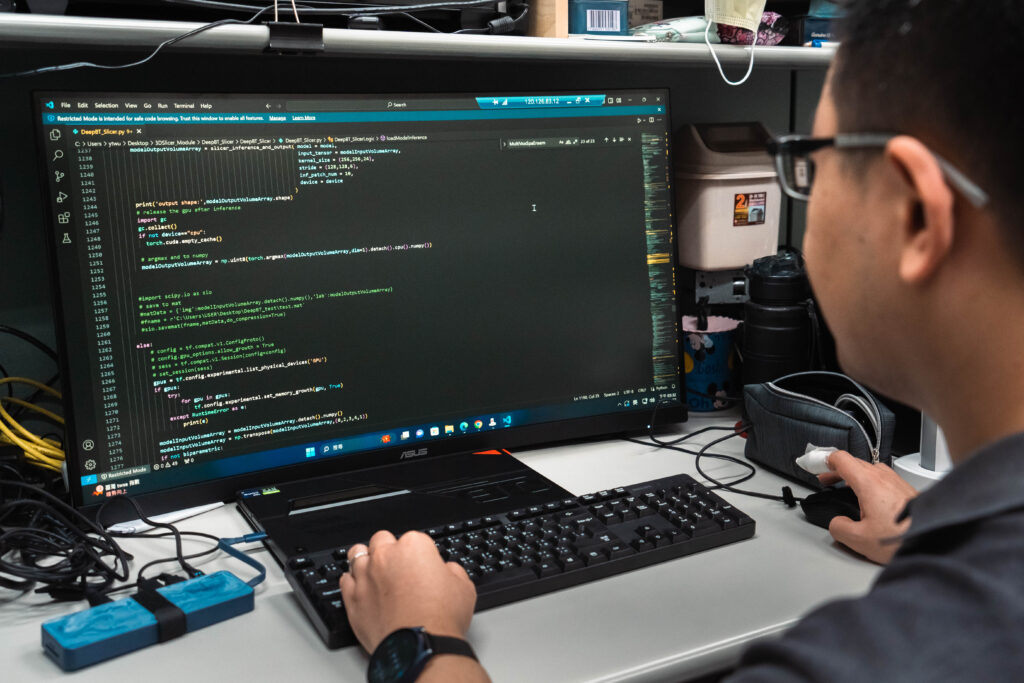
Dr. Wu observed that global academic and healthcare institutes have been actively developing AI detection and annotating tumor systems; however, the accuracy and quality for marking tumors are uneven due to only thousands of cases used for training AI models in many institutions. Taipei Veterans General Hospital was the first medical center in Taiwan that introduced the Gamma Knife and has accumulated up to millions of 2D MRI brain tumor images for dozens of years. In addition, those MRI images were jointly consulted and delineated by three physicians of the Division of Neuroradiology, Division of Radiation Oncology, and Division of Neurosurgery to ensure the consistency and objectiveness of interpretation results.
Dr. Wu. said, “Utilizing high quality and accurate annotations of tremendous data for AI model learning is our greatest competitiveness. Also, DeepBT™ is the one and only product whose model was trained by multi-parameter images!” Dr. Wu said supplementarily, “We combined two types of T1W+C and T2W parametric 3D images to overcome problems such as tumor contours, volumes, and positions that the traditional 2D images cannot handle. For the delineating effects on the solid or cystic tumors, our model is obviously superior to other models with the learning of one parametric images. The detective rate of the system is up to 94%, and the tumor contouring time shortens from 20 minutes to 1 minute, reducing 95% of the diagnostic time.”
After utilizing DeepBT™ to assist most of the brain tumor annotation tasks, physicians not only can focus on planning the treatment regime but also predict the prognosis by the system. For example, to predict whether the acoustic neuroma exists any “pseudoprogression” and the risk of edema after meningioma surgery, etc. “We have developed the new generation Detector+ model to follow up and annotate the pre-and post-operative tumors on multiple time points to objectively quantify data and assist physicians and patients to understand the change of the tumor volume and the number of tumors for treatment adjustment according to their therapeutic effectiveness,” said Dr. Wu.
Diligently Planned to Obtain Patents and Certifications and A Competitive Brain and Cardiopulmonary Testing
“AItewan DeepBT Detector” was awarded and recognized by “2022 FutureTech Award”, “2022 National Innovation Award”, “Excelsior Award of 2023 National Innovation Award” and certified and licensed Class II Medical Devices by Taiwan Food and Drug Administration (TFDA) in July 2023. AItewan DeepBT Detector was the first certificate of Class II Medical Devices for National Yang Ming Chiao Tung University and has been trialed in three medical centers in the northern and central regions. The second generation Detector+ model is estimated to be sent to TFDA for applying certification in the first quarter of 2024, and to the U.S. Food and Drug Administration for review in the third quarter of 2024.
Dr. Wu indicated, “We have received a total of four patents in three countries, Taiwan, Japan, and the U.S., and the seven patents in the European Union, China, and Southeast Asia have been applying.” Dr. Wu said that the clinical trials conducted by Taiwan’s major medical centers were basically recognized by Southeast Asia countries such as Thailand, the Philippines, and Vietnam, and only two to three months were needed to issue the local certification; hence, Southeast Asia countries and the U.S will be the next targeting markets that we planned to sell our products. In addition to brain tumors, we have planned to utilize and apply AI models to the detection of cardiovascular calcification, or fields such as low-dose Computed Tomography (LDCT) pulmonary nodules examination and follow-up. I believe we are highly competitive.
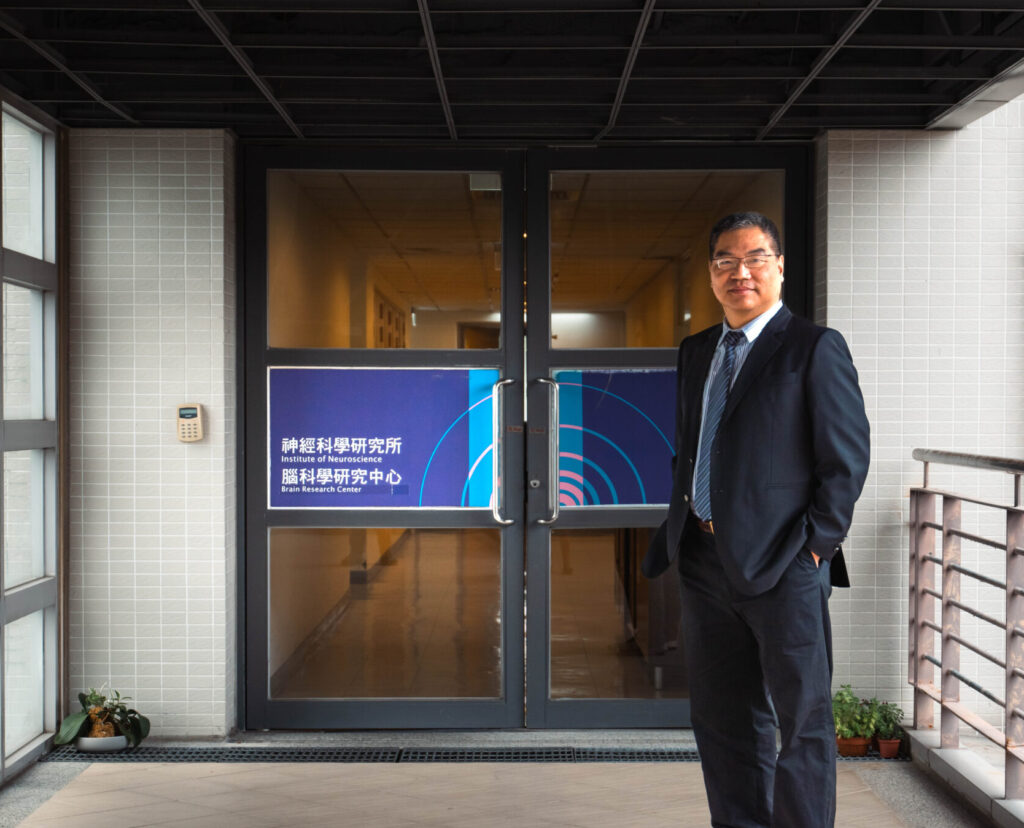
Finally, Dr. Wu considered that AI models have not only benefited in working flows such as testing, diagnosis, treatment, etc. for physicians but also the indistinctly observable developing trend of “generative AI” combined with medical treatments. “For example, all the medical histories from patients will be compiled and analyzed with AI to serve as the reference for the diagnosis by physicians, or lesions will be marked automatically by AI, and the results and predictions will be integrated into the physicians’ report system,” the ideal diagnosis situation depicted by Dr. Wu.
Dr. Wu said, “After patients have completed their examination, AI will rapidly generate a summarized written report. Absolutely, the physician who renders the report has to double-check. The next day when the patient calls the hospital, AI will be very likely to answer the call and the patient can communicate with AI to discuss and understand precautions. With timely feedback from AI, patients do not need to worry and wait for two weeks. Genuinely, this is what a thought ‘centralized on patients’ is like.” Dr. Wu optimistically said that we are all towards the development of a Large Language Model (LLM). Perhaps, AI communication timely with humans on healthcare occasions would be realized in the next five to ten years.
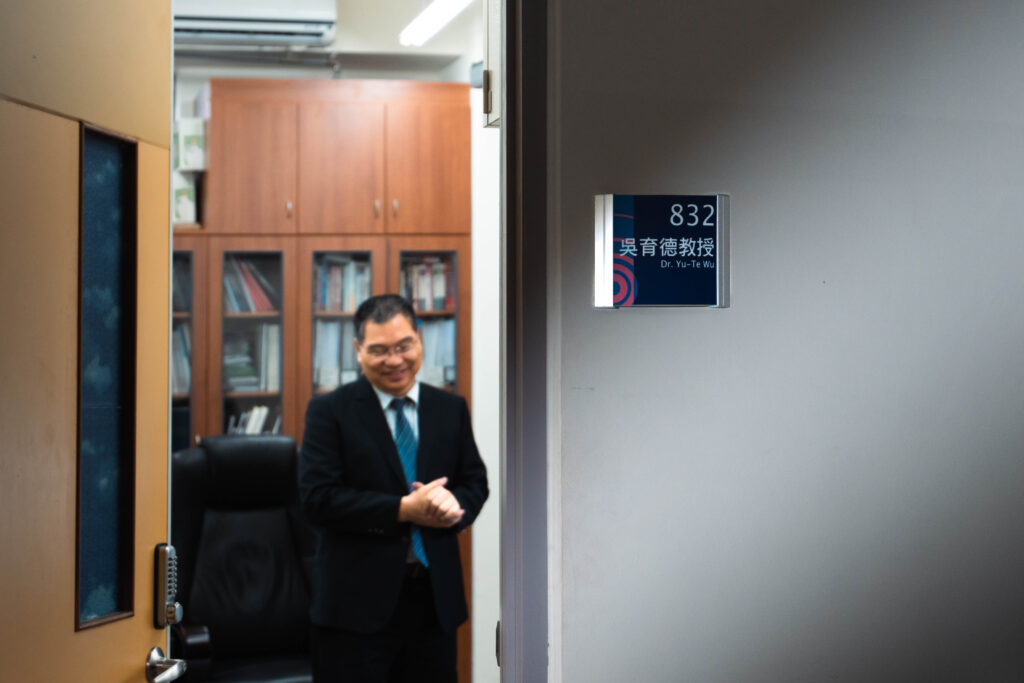